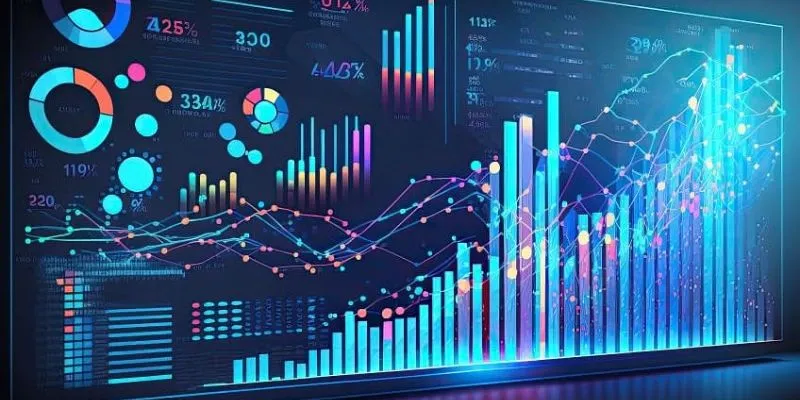
In today’s fast-paced digital world, businesses are generating massive amounts of data every single day. This is where data science comes into play. By leveraging the Data Science Course in Chennai, businesses can identify patterns, anticipate customer demands, and make informed decisions that drive growth.
In this blog, we’ll break down the key concepts, real-world applications, and techniques that make data-driven trend prediction possible.
What is Data Science, and Why is It Important for Business?
Data science is an interdisciplinary field that combines machine learning, programming, and domain expertise to extract valuable insights from raw data. Businesses use these insights to improve efficiency, enhance customer experiences.
With the right data science approach, businesses can:
- Understand customer behavior and preferences.
- Identify emerging market trends.
- Optimize operations and reduce costs.
- Improve decision-making with data-driven strategies.
- Enhance product recommendations and customer engagement.
Now, let’s dive into how businesses can use data science to predict trends and make proactive business decisions.
The Role of Data Science in Predicting Business Trends
1. Data Collection: The Foundation of Trend Prediction
Before predicting any trends, businesses need to gather relevant data. The accuracy of trend prediction heavily depends on the quality and quantity of data collected. Some common sources of business data include:
- Customer transactions and purchase history.
- Website traffic and user engagement metrics.
- Social media interactions and sentiment analysis.
- Industry reports and competitor analysis.
- Economic indicators and market trends.
By leveraging multiple Data Science Course in Bangalore, businesses can gain a comprehensive view of market dynamics and customer preferences.
2. Data Processing and Cleaning: Turning Raw Data into Valuable Insights
Raw data is often messy, incomplete, and inconsistent. Before applying any predictive models, businesses must clean and preprocess the data. This includes:
- Removing duplicate entries and inconsistencies.
- Handling missing values to prevent biased results.
- Standardizing data formats for uniform analysis.
- Eliminating irrelevant data points to improve model accuracy.
Once the data is cleaned, businesses can move forward with applying data science techniques to identify patterns and trends.
3. Identifying Patterns with Descriptive Analytics
Descriptive analytics helps businesses understand past and current trends by analyzing historical data. This involves:
- Identifying seasonal trends in sales and customer demand.
- Analyzing customer purchasing patterns.
- Detecting correlations between different business metrics.
- Using visualizations (graphs, charts, heatmaps) to interpret data more effectively.
By analyzing historical data, businesses can establish a foundation for predictive modeling and make better strategic decisions.
Advanced Techniques for Predicting Business Trends
4. Predictive Analytics: Forecasting the Future with Machine Learning
It plays an crucial role in various industries, helping businesses make data-driven decisions. If you’re looking to gain expertise in this field, enrolling in a Data Analytics Course in Chennai can provide hands-on experience with real-world data, equipping you with the skills needed to analyze trends and build predictive models effectively. Here are some of the most commonly used techniques:
A. Time Series Analysis
It is a technique used to predict future values based on past trends. Businesses use this approach to:
- Forecast sales revenue.
- Predict inventory demand.
- Analyze stock market trends.
- Optimize marketing campaigns.
Popular models used in time series forecasting include ARIMA (Auto-Regressive Integrated Moving Average) and LSTM (Long Short-Term Memory) neural networks.
B. Regression Analysis
These are used to determine relationships between variables. For example, a business might use regression analysis to predict how changes in marketing budget impact sales revenue.
C. Machine Learning Algorithms
Some common machine learning algorithms used for trend prediction include:
- Random Forest – Used for classifying data and predicting outcomes.
- Support Vector Machines (SVM) – Useful for identifying hidden patterns in data.
- Neural Networks – Effective in analyzing large-scale and complex datasets.
By implementing these models, businesses can accurately forecast customer demand, optimize pricing strategies, and predict shifts in the market.
5. Sentiment Analysis: Understanding Market Perception
Sentiment analysis helps businesses understand public opinion. To master this technique and other data analytics skills, enrolling in Data Analytics Training in Bangalore can be a great choice. This training provides hands-on experience in handling real-world data, equipping you with the knowledge to extract insights and make data-driven decisions effectively. It helps businesses:
- Gauge customer satisfaction and brand reputation.
- Identify potential PR crises before they escalate.
- Understand market demand for specific products or services.
- Tailor marketing campaigns based on customer sentiment.
6. Competitive Analysis: Staying Ahead of the Curve
Data science can help businesses analyze competitors and identify industry trends. By monitoring competitor pricing, marketing strategies, and customer engagement, businesses can:
- Adjust their own strategies for competitive advantage.
- Identify gaps in the market and emerging opportunities.
- Optimize their pricing methods to tempt more customers.
Using big data analytics and business intelligence tools, companies can make smarter decisions that help them stay ahead of competitors. However, as data-driven decision-making grows, it is essential to address Ethical Considerations in Data Science. Ensuring data privacy, avoiding bias in algorithms, and maintaining transparency in data usage are crucial aspects that organizations must focus on to build trust and make responsible business decisions.
Real-World Applications of Data Science in Business Trend Prediction
1. Retail Industry
Retailers use data science to:
- Predict customer buying behavior.
- Optimize inventory management.
- Personalize marketing campaigns based on past purchases.
2. Finance and Banking
Financial institutions leverage data science for:
- Fraud detection and risk assessment.
- Stock market trend prediction.
- Credit scoring and loan approvals.
3. Healthcare Industry
In healthcare, data science helps:
- Forecast disease outbreaks.
- Improve patient care with predictive analytics.
- Optimize hospital resource allocation.
4. E-Commerce and Digital Marketing
Online businesses use data science to:
- Recommend products based on browsing history.
- Optimize ad targeting using customer segmentation.
- Predict customer churn and improve retention strategies.
By leveraging predictive analytics, machine learning, and big data, companies can anticipate customer needs, optimize operations, and make data-driven decisions that drive growth. Among these technologies, Machine Learning Enhances Data Science by enabling automated pattern recognition, improving predictive accuracy, and uncovering valuable insights from complex datasets, making it an essential tool for modern businesses.